When AI plays favourites: How algorithmic bias shapes the hiring process
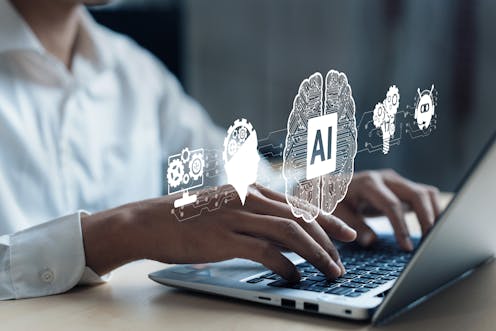
Given the rapid integration of AI into human resource management across many organizations, it’s important to raise awareness about the complex ethical challenges it presents. (Shutterstock)
A public interest group filed a U.S. federal complaint against artificial intelligence hiring tool, HireVue, in 2019 for deceptive hiring practices. The software, which has been adopted by hundreds of companies, favoured certain facial expressions, speaking styles and tones of voice, disproportionately disadvantaging minority candidates.
The Electronic Privacy Information Center argued HireVue’s results were “biased, unprovable and not replicable.” Though the company has since stopped using facial recognition, concerns remain about biases in other biometric data, such as speech patterns.
Similarly, Amazon stopped using its AI recruitment tool, as reported in 2018, after discovering it was biased against women. The algorithm, trained on male-dominated resumes submitted over 10 years, favoured male candidates by downgrading applications that included the word “women’s” and penalizing graduates of women’s colleges. Engineers tried to address these biases, but could not guarantee neutrality, leading to the project’s cancellation.
These examples highlight a growing concern in recruitment and selection: while some companies are using AI to remove human bias from hiring, it can often reinforce and amplify existing inequalities. Given the rapid integration of AI into human resource management across many organizations, it’s important to raise awareness about the complex ethical challenges it presents.
Ways AI can create bias
As companies increasingly rely on algorithms to make critical hiring decisions, it’s crucial to be aware of the following ways AI can create bias in hiring:
1. Bias in training data. AI systems rely on large datasets — referred to as training data — to learn patterns and make decisions, but their accuracy and fairness are only as good as the data they are trained on. If this data contains historical hiring biases that favour specific demographics, the AI will adopt and reproduce those same biases. Amazon’s AI tool, for example, was trained on resumes from a male-dominated industry, which led to gender bias.
2. Flawed data sampling. Flawed data sampling occurs when the dataset used to train an algorithm is not representative of the broader population it’s meant to serve. In the context of hiring, this can happen if training data over-represents certain groups —typically white men — while under-representing marginalized candidates.
As a result, the AI may learn to favour the characteristics and experiences of the over-represented group while penalizing or overlooking those from underrepresented groups. For example, facial analysis technologies have shown to have higher error rates for racialized individuals, particularly racialized women, because they are underrepresented in the data used to train these systems.
Read more:
Artificial intelligence can discriminate on the basis of race and gender, and also age
3. Bias in feature selection. When designing AI systems, developers choose certain features, attributes or characteristics to be prioritized or weighed more heavily when the AI is making decisions. But these selected features can lead to unfair, biased outcomes and perpetuate pre-existing inequalities.
For example, AI might disproportionately value graduates from prestigious universities, which have historically been attended by people from privileged backgrounds. Or, it might prioritize work experiences that are more common among certain demographics.
This problem is compounded when the features selected are proxies for protected characteristics, such as zip code, which can be strongly related to race and socioeconomic status due to historical housing segregation.
Bias in hiring algorithms raises serious ethical concerns and demands greater attention toward the mindful, responsible and inclusive use of AI.
(Shutterstock)
4. Lack of transparency. Many AI systems function as “black boxes,” meaning their decision-making processes are opaque. This lack of transparency makes it difficult for organizations to identify where bias might exist and how it affects hiring decisions.
Without insight into how an AI tool makes decisions, it’s difficult to correct biased outcomes or ensure fairness. Both Amazon and HireVue faced this issue; users and developers struggled to understand how the systems assessed candidates and why certain groups were excluded.
5. Lack of human oversight. While AI plays an important role in many decision-making processes, it should augment, rather than replace, human judgment. Over-reliance on AI without adequate human oversight can lead to unchecked biases. This problem is exacerbated when hiring professionals trust AI more than their own judgment, believing in the technology’s infallibility.
Overcoming algorithmic bias in hiring
To mitigate these issues, companies must adopt strategies that prioritize inclusivity and transparency in AI-driven hiring processes. Below are some key solutions for overcoming AI bias:
1. Diversify training data. One of the most effective ways to combat AI bias is to ensure training data is inclusive, diverse and representative of a wide range of candidates. This means including data from diverse racial, ethnic, gender, socioeconomic and educational backgrounds.
2. Conduct regular bias audits. Frequent and thorough audits of AI systems should be conducted to identify patterns of bias and discrimination. This includes examining the algorithm’s outputs, decision-making processes and its impact on different demographic groups.
It is important to actively involve human judgment in AI-driven decisions, particularly when making final hiring choices.
(Shutterstock)
3. Implement fairness-aware algorithms. Use AI software that incorporates fairness constraints and is designed to consider and mitigate bias by balancing outcomes for underrepresented groups. This can include integrating fairness metrics such as equal opportunity, modifying training data to show less bias and adjusting model predictions based on fairness criteria to increase equity.
4. Increase transparency. Seek AI solutions that offer insight into their algorithms and decision-making processes to make it easier to identify and address potential biases. Additionally, make sure to disclose any use of AI in the hiring process to candidates to maintain transparency with your job applicants and other stakeholders.
5. Maintain human oversight. To maintain control over hiring algorithms, managers and leaders must actively review AI-driven decisions, especially when making final hiring choices. Emerging research highlights the critical role of human oversight in safeguarding against the risks posed by AI applications. However, for this oversight to be effective and meaningful, leaders must ensure that ethical considerations are part of the hiring process and promote the responsible, inclusive and ethical use of AI.
Bias in hiring algorithms raises serious ethical concerns and demands greater attention toward the mindful, responsible and inclusive use of AI. Understanding and addressing the ethical considerations and biases of AI-driven hiring is essential to ensuring fairer hiring outcomes and preventing technology from reinforcing systemic bias.
Mehnaz Rafi does not work for, consult, own shares in or receive funding from any company or organisation that would benefit from this article, and has disclosed no relevant affiliations beyond their academic appointment.
Welcome to Billionaire Club Co LLC, your gateway to a brand-new social media experience! Sign up today and dive into over 10,000 fresh daily articles and videos curated just for your enjoyment. Enjoy the ad free experience, unlimited content interactions, and get that coveted blue check verification—all for just $1 a month!
Account Frozen
Your account is frozen. You can still view content but cannot interact with it.
Please go to your settings to update your account status.
Open Profile Settings