I was a beta tester for the Nobel prize-winning AlphaFold AI – it’s going to revolutionise health research
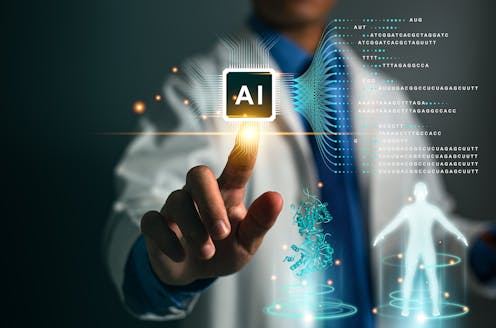
S. Singha/ShutterstockThe deep learning machine AlphaFold, which was created by Google’s AI research lab DeepMind, is already transforming our understanding of the molecular biology that underpins health and disease.
One half of the 2024 Nobel prize in chemistry went to David Baker from the University of Washington in the US, with the other half jointly awarded to Demis Hassabis and John M. Jumper, both from London-based Google DeepMind.
If you haven’t heard of AlphaFold, it may be difficult to appreciate how important it is becoming to researchers. But as a beta tester for the software, I got to see first-hand how this technology can reveal the molecular structures of different proteins in minutes. It would take researchers months or even years to unpick these structures in laboratory experiments.
Read more:
Google Deepmind founder shares Nobel prize in chemistry for AI that unlocks the shape of proteins
This technology could pave the way for revolutionary new treatments and drugs. But first, it’s important to understand what AlphaFold does.
Proteins are produced by series of molecular “beads”, created from a selection of the human body’s 20 different amino acids. These beads form a long chain that folds up into a mechanical shape that is crucial for the protein’s function.
Their sequence is determined by DNA. And while DNA research means we know the order of the beads that build most proteins, it’s always been a challenge to predict how the chain folds up into each “3D machine”.
Nobel prize laureates David Baker, Demis Hassabis and John M. Jumper.
Illustrations: Niklas Elmehed © Nobel Prize Outreach, CC BY-NC-SA
These protein structures underpin all of biology. Scientists study them in the same way you might take a clock apart to understand how it works. Comprehend the parts and put together the whole: it’s the same with the human body.
Proteins are tiny, with a huge number located inside each of our 30 trillion cells. This meant for decades, the only way to find out their shape was through laborious experimental methods – studies that could take years.
Throughout my career I, along with many other scientists, have been engaged in such pursuits. Every time we solve a protein structure, we deposit it in a global database called the Protein Data Bank, which is free for anyone to use.
AlphaFold was trained on these structures, the majority of which were found using X-ray crystallography. For this technique, proteins are tested under thousands of different chemical states, with variations in temperature, density and pH. Researchers use a microscope to identify the conditions under which each protein lines up in a particular formation. These are then shot with X-rays to work out the spatial arrangement of all the atoms in that protein.
Having been trained on these structures, AlphaFold can now predict protein structure at speeds that were previously impossible.
I started out early in my career, from the late 90s, working out protein structures using magnetic properties of their nuclei. I did this with technology called nuclear magnetic resonance (NMR) spectroscopy, which uses a huge magnet like an MRI scanner. This method had begun to fall out of favour because of certain technical limitations, but is now having a resurgence thanks to AlphaFold.
NMR is one of the few techniques that can probe molecules in motion, instead of keeping them still inside a crystal or on an electron microscope grid.
Addictive experience
In March 2024, researchers at DeepMind approached me to beta test AlphaFold3, the latest incarnation of the software, which was close to release at the time.
I’ve never been a gamer but I got a taste of the addictive experience as, once I got access, all I wanted to do was spend hours trying out molecular combinations. As well as lightning speed, this new version introduced the option to include bigger and more varied molecules, including DNA and metals, and the opportunity to modify amino acids to mimic chemical signalling in cells.
Our lab at King’s College London used X-ray crystallography to predict a structure formed by two bacterial proteins that are loosely involved in hospital superbugs when they interact. Previous incarnations of AlphaFold predicted the individual components but could never get the complex right – yet this new version solved it at the first attempt.
Understanding the moving parts and dynamics of proteins is the next frontier, now that we can predict static protein shapes with AlphaFold. Proteins come in a huge variety of shapes and sizes. They can be rigid or flexible, or made of neatly structured units connected by bendy loops.
Dynamics are essential for protein function. As another Nobel laureate, Richard Feynman, said: “Everything that living things do can be understood in terms of the jiggling and wiggling of atoms.”
Another great feature of magnetic resonance techniques is they can measure precise distances between atoms. So, with a few carefully designed experiments, the AlphaFold outputs can be verified in a lab.
In other cases, the results are still ambiguous. It’s a work in progress between experimental structural biologists, like my team, and computational scientists.
The recognition that comes with a Nobel prize will only galvanise the quest to understand all molecular machinery – and hopefully, change the game when it comes to medicines, vaccines and human health.
Rivka Isaacson receives funding from the UK Research and Innovation Biotechnology and Biological Sciences Research Council.
Welcome to Billionaire Club Co LLC, your gateway to a brand-new social media experience! Sign up today and dive into over 10,000 fresh daily articles and videos curated just for your enjoyment. Enjoy the ad free experience, unlimited content interactions, and get that coveted blue check verification—all for just $1 a month!
Account Frozen
Your account is frozen. You can still view content but cannot interact with it.
Please go to your settings to update your account status.
Open Profile Settings