From MOCO v1 to v3: Towards Building a Dynamic Dictionary for Self-Supervised Learning — Part 1
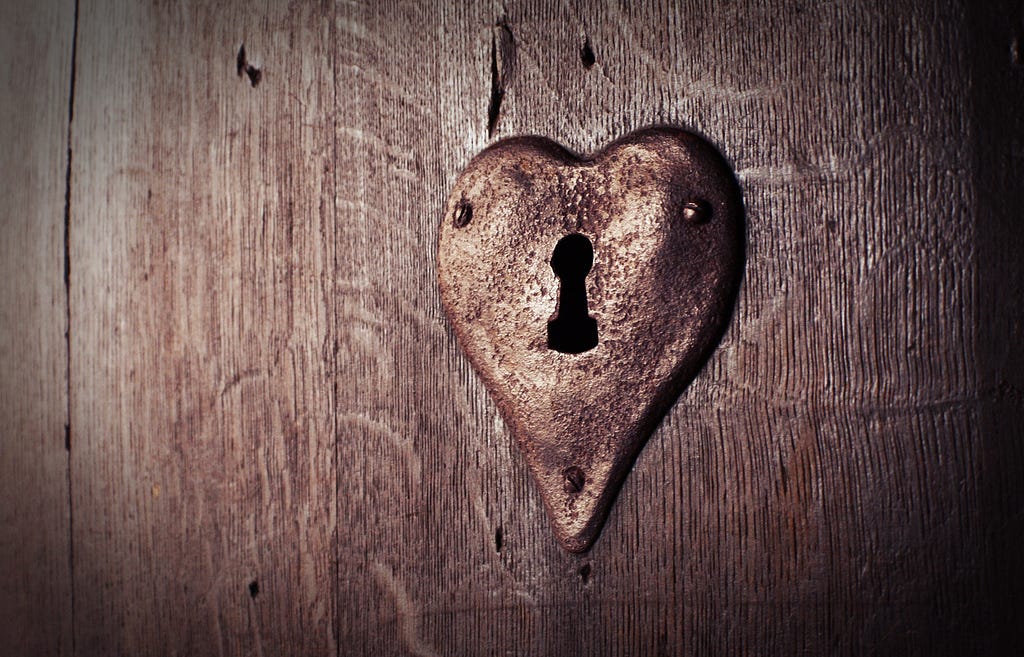
From MOCO v1 to v3: Towards Building a Dynamic Dictionary for Self-Supervised Learning — Part 1A gentle recap on the momentum contrast learning frameworkHave we reached the era of self-supervised learning?Data is flowing in every day. People are working 24/7. Jobs are distributed to every corner of the world. But still, so much data is left unannotated, waiting for the possible use by a new model, a new training, or a new upgrade.Or, it will never happen. It will never happen when the world is running in a supervised fashion.The rise of self-supervised learning in recent years has unveiled a new direction. Instead of creating annotations for all tasks, self-supervised learning breaks tasks into pretext/pre-training (see my previous post on pre-training here) tasks and downstream tasks. The pretext tasks focus on extracting representative features from the whole dataset without the guidance of any ground truth annotations. Still, this task requires labels generated automatically from the dataset, usually by extensive data augmentation. Hence, we use the terminologies unsupervised learning (dataset is unannotated) and self-supervised learning (tasks are supervised by self-generated labels) interchangeably in this article.Contrastive learning is a major category of self-supervised learning. It uses unlabelled datasets and contrastive information-encoded losses (e.g., contrastive loss, InfoNCE loss, triplet loss, etc.) to train the deep learning network. Major contrastive learning includes SimCLR, SimSiam, and the MOCO series.MOCO — the word is an abbreviation for “momentum contrast.” The core idea was written in the first MOCO paper, suggesting the understanding of a computer vision self-supervised learning problem, as follows:“[quote from original paper] Computer vision, in contrast, further concerns dictionary building, as the raw signal is in a continuous, high-dimensional space and is not structured for human communication… Though driven by various motivations, these (note: recent visual representation learning) methods can be thought of as building dynamic dictionaries… Unsupervised learning trains encoders to perform dictionary look-up: an encoded ‘query’ should be similar to its matching key and dissimilar to others. Learning is formulated as minimizing a contrastive loss.”In this article, we’ll do a gentle review of MOCO v1 to v3:v1 — the paper “Momentum contrast for unsupervised visual representation learning” was published in CVPR 2020. The paper proposes a momentum update to key ResNet encoders using sample queues with InfoNCE loss.v2 — the paper “ Improved baselines with momentum contrastive learning” came out immediately after, implementing two SimCLR architecture improvements: a) replacing the FC layer with a 2-layer MLP and b) extending the original data augmentation by including blur.v3 — the paper “An empirical study of training self-supervised vision transformers” was published in ICCV 2021. The framework extends the key-query pair to two key-query pairs, which were used to form a SimSiam-style symmetric contrastive loss. The backbone also got extended from ResNet-only to both ResNet and ViT.Image source: https://pxhere.com/en/photo/760197MOCO V1The framework starts at a core self-supervised learning concept: query and keys. Here, query refers to the representation vector of the query image or patches (x^query), while keys refer to the representation vectors of the sample image/patch dictionaries ({x_0^key, x_1^key, …}). The query vector q is generated by a trainable “main” encoder with regular gradient backpropagation. The key vectors, stored in a dictionary queue, are generated by a trainable encoder, which doesn’t do gradient backpropagation directly but only updates the weights in a momentum fashion using the main encoder’s weights. See the update style below:Image source: https://arxiv.org/pdf/1911.05722Instance discrimination task and InfoNCE lossA specific task is needed since the dataset does not have labels at the pretext/pre-training stage. The paper adopted the instance discrimination task proposed in this CVPR 2018 paper. Unlike the original design, where the similarity between feature vectors in the memory bank was calculated using a non-parametric classifier, the MOCO paper used the positive +<query, key> pair and negative -<query, key> pair to supervise the learning process. A pair is considered positive when the query and key image are augmented from the same image. Otherwise, it is negative. The training loss is the InfoNCE loss, which can be considered as the negative logarithm of the softmax of the query/key pairs:Equation source: https://arxiv.org/pdf/1911.05722Momentum updateThe authors claim that copying the main query encoder to the key encoder would likely cause poor results because a rapidly changing encoder will reduce the key representation dictionary’s consistency. Instead, only the main query encoder is trained at each step, but the weights of the key encoder are updated using a momentum weight m:Equation source: https://arxiv.org/pdf/1911.05722The momentum weight is kept large during the training, e.g., 0.999 rather than 0.9, which validates the authors’ guess that the key encoder’s consistency and stability affect the contrastive learning performance.PseudocodeThe less-than-20-lines pseudo code is a quick outline of the whole training process. Consistent with the InfoLoss shown above, it is worth noting that the positive logit is a single scale per sample, and the negative logit is a K-element vector per sample corresponding to the K keys.Pseudocode from: https://arxiv.org/pdf/1911.05722MOCO V2Released immediately after MOCO, the 2-page v2 paper proposed minor changes to version 1 by adopting two successful architecture changes from SimCLR:replacing the fully connected layer of the ResNet encoder with a 2-layer MLPextending the original augmentation set with blur augmentationInterestingly, even with one simple architecture tweak, the performance boost seems significant:Image source: https://arxiv.org/pdf/2003.04297MOCO V3Version 3 proposed major improvements by adopting a symmetric contrastive loss, extra projection head, and ViT encoder.Symmetric contrastive lossInspired by the SimSiam work, which takes two randomly augmented views and switches them in the negative cosine similarity computation to obtain a symmetric loss, MOCO v3 augments the sample twice. It feeds them separately to the query and key encoders.Image source: https://arxiv.org/pdf/1911.05722 and https://arxiv.org/abs/2104.02057The symmetric contrastive loss is based on the simple assumption — all positive pairs are in the diagonal of the N*N query-key matrix since they are the augmentations of the same image; all negative pairs are on other locations of the N*N query-key matrix as they are augmentations (might be the same augmentation) from different samples:Image source: https://arxiv.org/abs/2104.02057In this sense, the dynamic key dictionary is much simpler as it’s calculated on the fly within the minibatch and doesn’t need to keep a memory queue. This can be validated by the stability analysis over batch sizes below (note the authors explained that the 6144-batch performance decreased because of the partial failure phenomenon during training time):Image source: https://arxiv.org/pdf/2104.02057ViT encoderThe performance boost by the ViT encoder is shown below:Image source: https://arxiv.org/pdf/2104.02057Comparisons and summaryThe MOCO v3 paper gives a performance comparison among v1-v3 using ResNet50 (R50) encoder:Image source: https://arxiv.org/pdf/2104.02057In summary, MOCO v1-v3 gave a clear transformation of the following elements:Encoder: ResNet → ResNet + MLP layers → ResNet/ViT + MLP layersKey dictionary: global key vector queues → minibatch keys based on augmentationContrastive loss: asymmetric contrastive loss → symmetric contrastive lossBut there is more. In the next article, I will dive deep into the MOCO v3 code to implement data augmentation and the momentum update. Stay tuned!ReferencesWu et al., Unsupervised feature learning via non-parametric instance discrimination. CVPR 2018. github: https://github.com/zhirongw/lemniscate.pytorchOord et al., Representation learning with contrastive predictive coding. arXiv preprint 2018.Chen et al., A simple framework for contrastive learning of visual representations. PMLR 2020. github: https://github.com/sthalles/SimCLRHe et al., Momentum contrast for unsupervised visual representation learning. CVPR 2020.Chen et al., Improved baselines with momentum contrastive learning. arXiv preprint 2020.Chen et al., Exploring simple siamese representation learning. CVPR 2021. github: https://github.com/facebookresearch/simsiamChen et al., An Empirical Study of Training Self-Supervised Vision Transformers. ICCV 2021. github: https://github.com/facebookresearch/moco-v3From MOCO v1 to v3: Towards Building a Dynamic Dictionary for Self-Supervised Learning — Part 1 was originally published in Towards Data Science on Medium, where people are continuing the conversation by highlighting and responding to this story.
Welcome to Billionaire Club Co LLC, your gateway to a brand-new social media experience! Sign up today and dive into over 10,000 fresh daily articles and videos curated just for your enjoyment. Enjoy the ad free experience, unlimited content interactions, and get that coveted blue check verification—all for just $1 a month!
Account Frozen
Your account is frozen. You can still view content but cannot interact with it.
Please go to your settings to update your account status.
Open Profile Settings